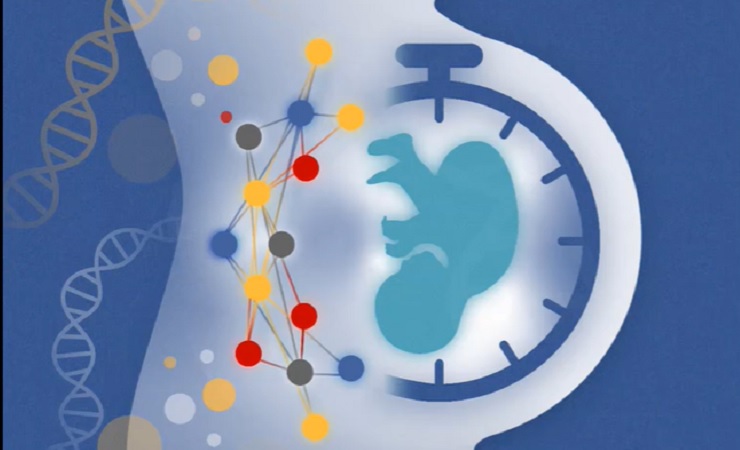
A scientific crowdsourcing initiative led by researchers of the Wayne State University Perinatal Initiative and the National Institutes of Health’s Perinatology Research Branch at the Wayne State University School of Medicine has developed promising predictive models for gestational age and spontaneous preterm birth.
“Crowdsourcing assessment of maternal blood multi-omics for predicting gestational age and preterm birth” published today June 15 in Cell Reports Medicine, details the methods and findings of the DREAM Preterm Birth Prediction Challenge launched in 2019 by WSU, the PRB and collaborators from several institutions.
The initiative invited researchers around the world to use maternal blood transcriptomics and proteomics data generated by the PRB and WSU to develop an accurate molecular clock of pregnancy and models to identify women at risk for preterm birth.
The challenge sought to bring the obstetrics and machine-learning community together to determine the value of maternal whole blood transcriptomics data for gestational dating and determining the risk of preterm birth. The ultimate goal was to identify, through big-data analytical approaches, a set of biomarkers that can be pursued in further targeted studies to develop convenient and affordable tests for pregnancy dating, and to identify patients at risk of preterm birth who can benefit from closer surveillance and treatment.
Harnessing the wisdom of scientists around the world, via a DREAM challenge, Adi Tarca, Ph.D., professor of Obstetrics and Gynecology, and adjunct professor of Computer Science at Wayne State University; Roberto Romero, M.D., D.Med.Sci., chief of the Perinatology Research Branch, program director for Perinatal Research and Obstetrics, Intramural Division of the Eunice Kennedy Shriver National Institute of Child Health and Human Development, together with co-authors from other institutions, developed methods to predict gestational age and preterm birth from longitudinal multi-omics data. They found that certain ribonucleic acids, or RNAs, predict ultrasound-based gestational age and identified molecular changes that precede a diagnosis of spontaneous preterm birth in asymptomatic women.
The DREAM challenges framework used in the study was pioneered by Gustavo Stolovitzky, Ph.D., program director of the Translational Systems Biology and Nanobiotechnology Program at IBM’s Thomas J. Watson Research Center. DREAM challenges were shown to make a difference in other areas of biomedicine by providing a robust evaluation of models and biodata. The DREAM challenge director overseeing the implementation of the challenge and senior author was James Costello, Ph.D.., of the Department of Pharmacology at the University of Colorado Anschutz Medical Campus.
“This study demonstrated evidence of superior performance by plasma proteomics compared to whole-blood transcriptomics in the prediction of spontaneous preterm delivery,” Dr. Tarca said. “The plasma proteomics signatures of spontaneous preterm birth identified here have direct implications for the development of future tests demonstrated in other health applications by combining reproducible proteomic signals with machine learning.”
More than 500 researchers worldwide took part in the challenge. The resulting computational algorithms are available to the scientific community via an open-source license, allowing for research and applications in similar research initiatives.
“The DREAM challenge is a fantastic opportunity to engage computational scientists in an important biomedical question. Leveraging data repositories such as the March of Dimes Database for Preterm Birth Research (https://pretermbirthdb.org/mod/home) in combination with crowdsourcing computational knowledge allows us to creatively tackle challenging research problems such as preterm birth,” said Marina Sirota, Ph.D., associate professor of Bakar Computational Health Sciences Institute at the University of California, San Francisco. “This is hopefully a first of many challenges leveraging diverse molecular data and advanced computational techniques that will be able to impact diagnostic and therapeutic strategies for preterm birth.”
The rate of preterm birth in the United States remains fundamentally unchanged in recent years, causing an annual societal economic burden of at least $26.2 billion. Worldwide, preterm birth causes more than 1 million deaths annually. Children who do survive preterm birth are at risk for a number of short- and long-term complications.